By: Hossein Mohammadian Foroushani, Ali Hamzehloo, Atul Kumar, Yasheng Chen, Laura Heitsch,
Agnieszka Slowik, Daniel Strbian, Jin-Moo Lee, Daniel S. Marcus & Rajat Dhar
First Online: 20 August 2021
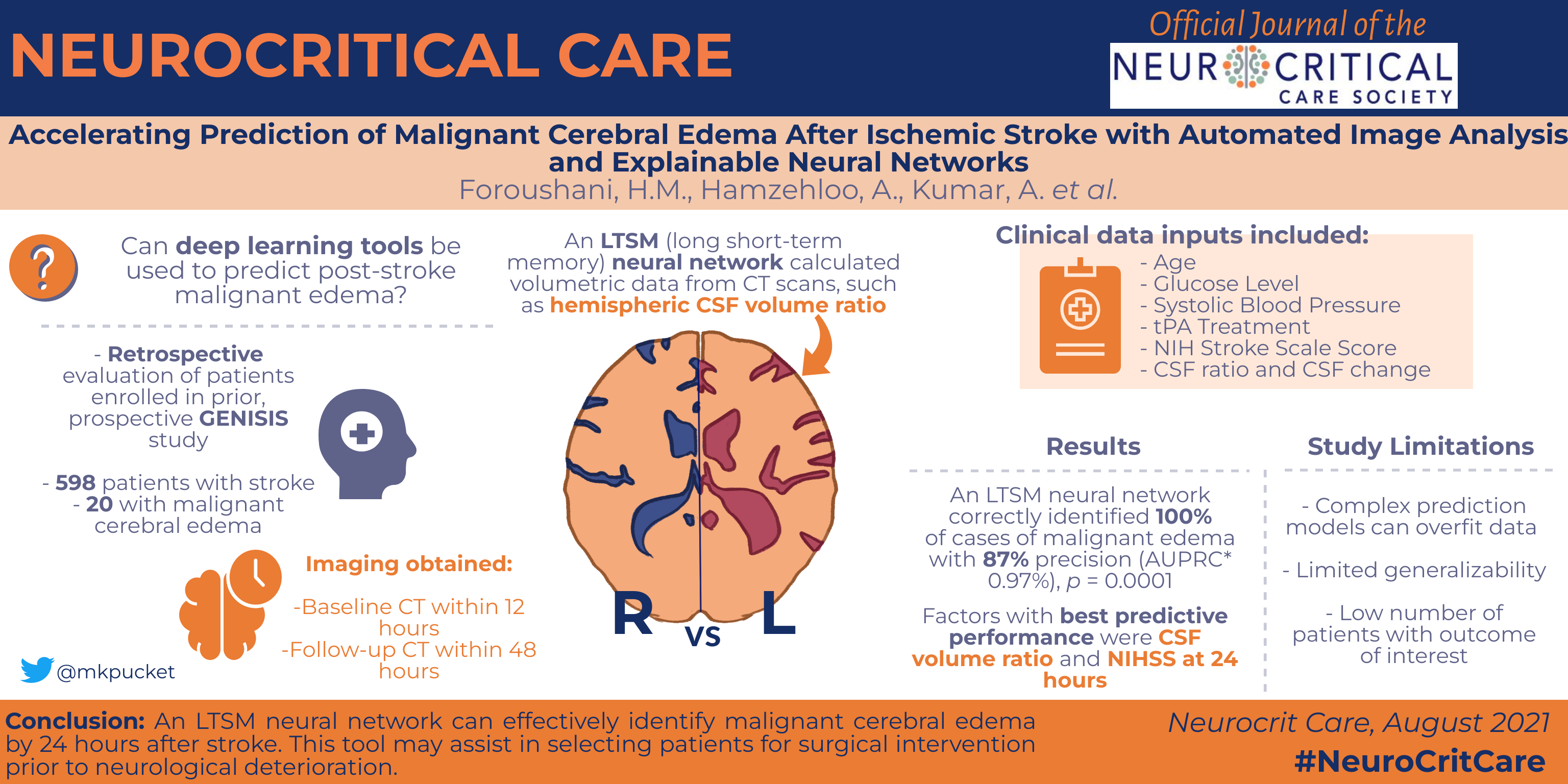
Background
Malignant cerebral edema is a devastating complication of stroke, resulting in deterioration and death if
hemicraniectomy is not performed prior to herniation. Current approaches for predicting this relatively rare
complication often require advanced imaging and still suffer from suboptimal performance. We performed a pilot study
to evaluate whether neural networks incorporating data extracted from routine computed tomography (CT) imaging could
enhance prediction of edema in a large diverse stroke cohort.
Methods
An automated imaging pipeline retrospectively extracted volumetric data, including cerebrospinal fluid (CSF) volumes
and the hemispheric CSF volume ratio, from baseline and 24 h CT scans performed in participants of an
international stroke cohort study. Fully connected and long short-term memory (LSTM) neural networks were trained
using serial clinical and imaging data to predict those who would require hemicraniectomy or die with midline shift.
The performance of these models was tested, in comparison with regression models and the Enhanced Detection of Edema
in Malignant Anterior Circulation Stroke (EDEMA) score, using cross-validation to construct precision-recall
curves.
Read the full article